From Data to Revenue: How Customer Potentialization Can Supercharge Sales
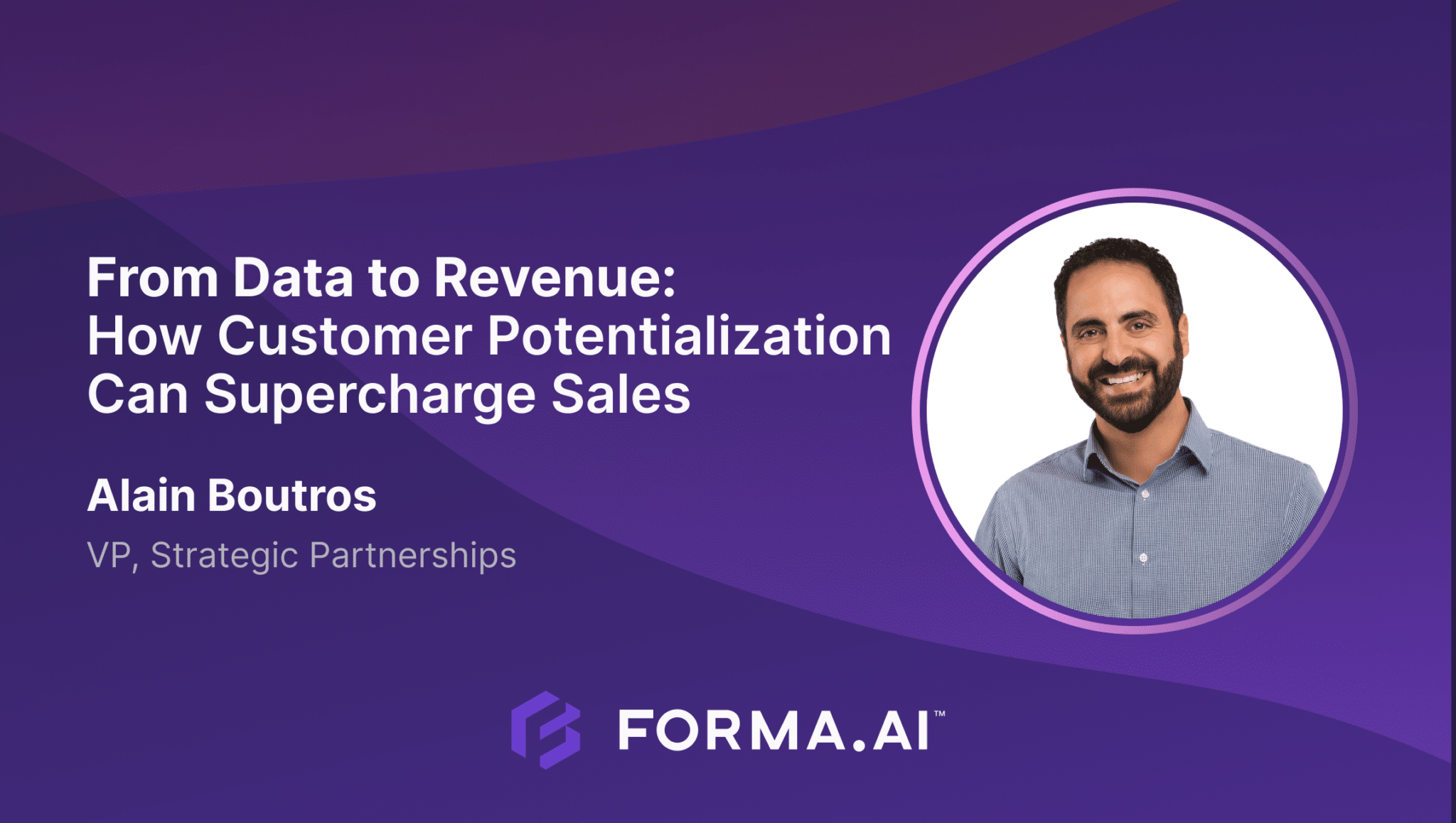
Customer potentialization helps enterprises identify and capitalize on customer value by deploying resources more effectively and efficiently.
It's one of the strategies we employ to help our enterprise clients expand into new markets and existing accounts.
By the end of this article, you will understand how to use customer potentialization to maximize customer value for your organization.
What is customer potentialization modeling?
Without granular market share data, customer potentialization helps quantify the total addressable market for a business and its growth opportunity. It allows companies to make better resource investment decisions and drive more predictable outcomes. Ultimately this analysis becomes a cornerstone of the Go-to-Market strategy.
A customer potentialization model is a data model that leverages account profile information and historical account performance to quantify what all accounts, existing and prospects, could spend on a company's products.
Customer potentialization models enable leadership to focus resources on capturing revenue from the highest value accounts, the value being derived from what that account can potentially spend with you and your current share of wallet with them.
We use customer potentialization models to ensure our customers deploy sales incentives more effectively by allocating quotas at a territory level based on historical performance and growth opportunities within each territory.
It's unfair to say all territories should grow by the same percentage yearly to get us to the national forecast. Instead, we should determine quotas based on where the real opportunity lies. Expected growth in under-penetrated territories should be higher than in territories you have been historically highly successful in and have a higher market share.
How to Build a Customer Potentialization Model
We build our customer potentialization models in five stages:
- Exploring data to identify account profile information correlated with historical spend.
- Segmenting accounts (existing customers and prospects) into clusters of accounts that look and behave the same.
- Identifying product-level frontier performance within each cluster that other accounts could achieve.
- Extrapolating the frontier performance to all accounts.
- Adding up product-level potential across accounts and products.
It seems simple enough, but there's complexity and rigor required to identify the correct variables, clusters, and appropriate frontier performance to create an effective model.
These models are further calibrated based on market estimates and as new sales performance data comes into play.
Related article: How to Maximize Sales Performance With Better Balanced Territories
Building a Customer Potentialization Model Step-By-Step
Now that you have the blueprint for customer potentialization modeling, let's dive into those steps in detail.
To allow us to get into specifics about the process, we'll use the example of one of our customers, a large B2B SaaS technology enterprise with a per-seat pricing model.
This enterprise has multiple complimentary products for a range of business applications. Their customers vary in size and the number of products they use, creating a lot of variances in the amount of revenue they produce and the workload required to service each account.
To enable leadership to identify opportunities better and allocate resources to expansion efforts, we constructed a customer potentialization model by identifying which factors correlated closest with revenue generation, measured as Annual Recurring Revenue (ARR) per user seat.
1. Exploring and analyzing historical customer data
The aim of this stage is to find which data points correlate closest with historical customer spend.
Be warned: The most relevant data for your business may not be the metrics you expect.
The entirety of your customer potentialization rests on this data, so take the time to validate the correlation before moving on to the next stage.
"What gets measured gets managed — even when it's pointless to measure and manage it, and even if it harms the purpose of the organization to do so."
V. F. Ridgway
We suggest starting with firmographic data such as size, revenue, location, industry, etc. In our customer's case, the following dimensions showed a high correlation with per-seat ARR and were included in the model:
- Customer employees
- Customer revenue
- Length of product use
We analyzed several other dimensions, including location, rural vs. urban areas, industry, and purchase history but excluded them from the model due to low correlation.
2. Segment your customers into clusters
The aim of this stage is to segment customers into roughly equally sized groups based on their historical buying patterns and usage behaviors.
In our customer example, the thresholds were based on correlation analysis and set to ensure a sufficient sample size of accounts in each cluster. The groups were based on their employee size and layering on products they used, length of usage, and average spend per employee.
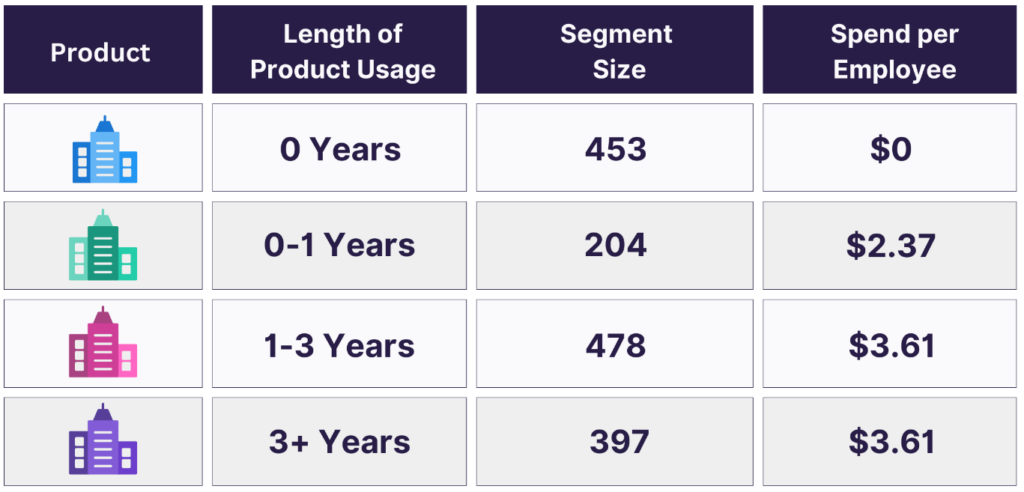
Add as many additional informational layers to your customer clusters as is appropriate for your product or service.
3. Identify Frontier Performance
Once you have established your clusters, ensuring they are fairly distributed and representative, identify the target spend level for each segment and product category. We do that by extrapolating the potential ARR-per-employee for each cluster based on the frontier performance of the preceding customer subgroup.
The graph below shows an example breakdown for a single subgroup of customers. Once we have distributed all customers in the subgroup across the x-axis, the target revenue for the subset is derived from around the 80-90th percentile on the curve. That prevents estimates from skewing towards outliers while still providing an ambitious and attainable target.
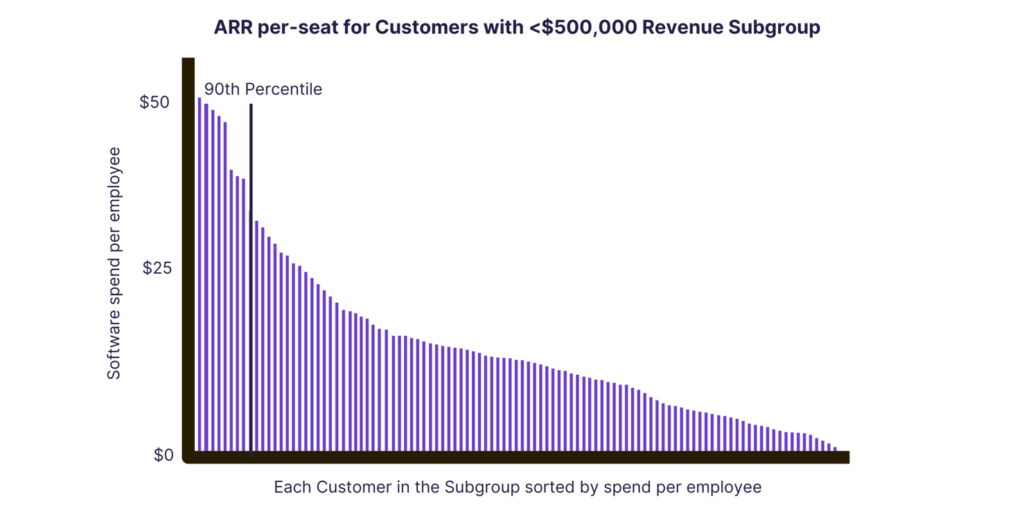
4. Extrapolate to Model Overall Customer Potential
With a target spend established, we can quickly identify underperforming or outperforming customers, particularly outliers, and set future goals by rolling the spend level of your most established customers back down across your segments.
In the case of our customer, that meant the frontier spend level for customers in their 3rd year of using each product was set as the future revenue target for customers in their second year of usage.
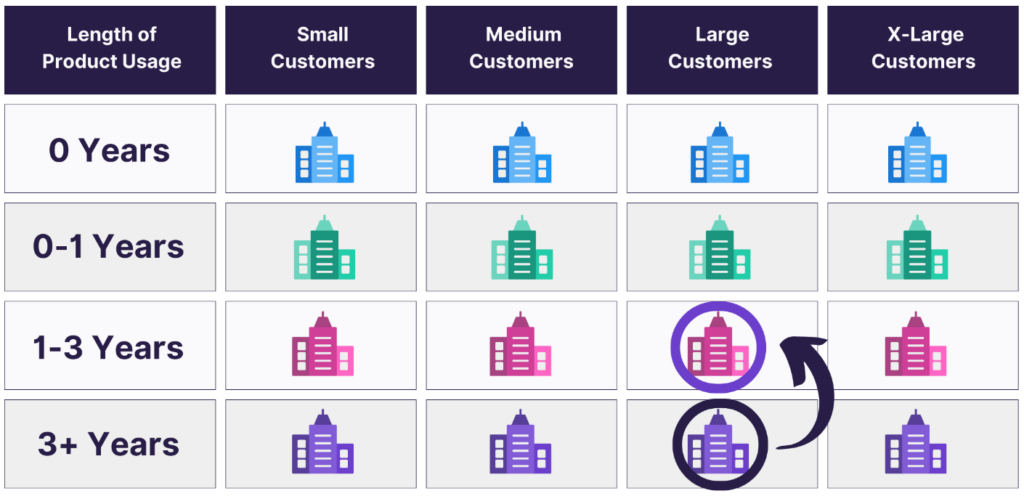
5. Add up product potential across accounts
After setting those figures at the subgroup level, we determined account-level potential by summing all the product potential estimates and multiplying by the employee headcount. That allows us to calculate the untapped potential of the total addressable market and identify growth opportunities.
The final dataset looks something like this:
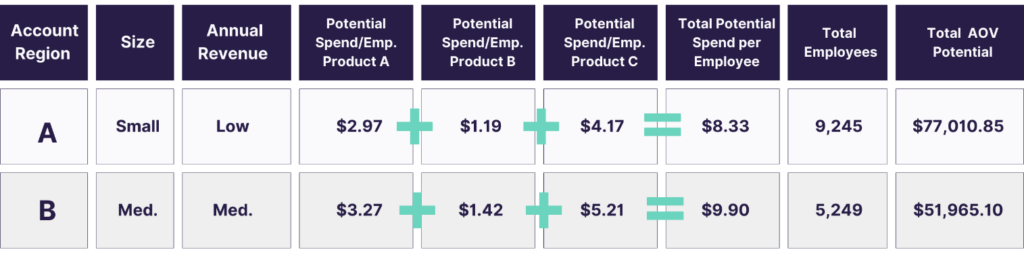
It's important to remember that this is an approximation. It will take time to refine and calibrate your initial model to reflect the circumstances on the ground as you learn more about how customer characteristics relate to buying behavior.
Validating and Refining Your Customer Potential Model
The macro-environment and customer behavior change over time, which will impact the accuracy and relevance of your model.
Inaccurate and outdated models lead to missed opportunities and inefficient resource allocation, as they do not accurately reflect the current business environment and customer behavior.
💡 REMEMBER: It is not possible to get customer potentialization models 100% accurate at the account level. They are designed to be used in aggregate to enable data-informed decisions such as territory design and quota setting.
To keep your customer potentialization model relevant and applicable, continually analyze behavioral and transactional data to identify customer buying patterns and preferences changes. This information can be used to adjust the model to reflect the current business environment and customer behavior accurately.
For example, you may observe a significant increase in the average purchase value for one product in a group of low-value customers. This change in customer behavior could indicate that the low-value customers in that cluster are now more valuable to the business and should be reclassified as mid-value or high-value customers. If you can identify what caused the change (if anything), you can then work to realize that potential in other similar customers.
Refining the customer value model to reflect this change can help the business prioritize efforts to retain and grow these valuable customers over time.
Related article: How to Unlock the Power of MBOs for Sales Compensation Success
Summary
Customer potentialization helps organizations identify and capitalize on customer value by quantifying the total addressable market for their business. It's an incredibly potent tool to inform targeting strategy, territory design, and quota setting.
By leveraging account profile information and historical transactional data, enterprises can focus resources on capturing revenue from the highest-value accounts and make better resource investment decisions.
With regular adjustment and validation, customer potentialization modeling can become a cornerstone of your go-to-market strategy, enabling leadership to allocate resources to expansion efforts effectively and efficiently, driving better outcomes for individual salespeople and the business overall.
Bring Customer Potentialization to Your Business
If your organization has attempted and failed to capitalize on customer potential, or if you have engaged a consultancy to develop your customer potentialization initiative but have been unable to maintain it, Forma.ai can help.
Advanced analytical modeling with ongoing maintenance is at the core part of our platform. Book a call here with one of our sales compensation experts to find out how to bring customer potentialization modeling — and more data-driven go-to-market strategies — to your business.